Table of Contents
Related pages
See also
Introduction
Labour income is the amount that employed people earn by working. Economists use this concept to distinguish it from capital income. Owners of assets earn capital income due to their property. Assets include land, machines, buildings or patents. Labour income includes the wages of employees and part of the income of the self-employed. Self-employed workers earn from both their work and capital ownership.
For many, their work is a key source of income. In contrast, studies show that capital income disproportionately benefits the affluent. Therefore, labour income data can bring new insights to understanding inequality. Furthermore, topics like automation, the gig economy, or globalisation have a strong link with labour income statistics.
Data catalogue
Indicator | Frequency | Database | Subject | Download (with labels) | Download (with codes) | Data explorer |
---|---|---|---|---|---|---|
SDG indicator 10.4.1 - Labour income share as a percent of GDP (%) | Annual | SDG Labour Market Indicators (ILOSDG) | Labour income | .csv .dta .xlsx | .csv.gz | |
Labour income share as a percent of GDP -- ILO modelled estimates, Aug. 2024 (%) | Annual | ILO Modelled Estimates (ILOEST) | Labour income | .csv .dta .xlsx | .csv.gz | |
Labour income distribution -- ILO modelled estimates, Nov. 2022 (%) | Annual | ILO Modelled Estimates (ILOEST) | Labour income | .csv .dta .xlsx | .csv.gz | |
Gender income gap, ratio of women's to men's labour income -- ILO modelled estimates, Aug. 2024 (%) | Annual | ILO Modelled Estimates (ILOEST) | Labour income | .csv .dta .xlsx | .csv.gz |
Latest posts
F.A.Q.
Labour income is any amount earned through working. Economists use it as a contraposition to capital income, which is the amount that capital owners earn through the utilisation of their capital. Capital includes any property used in production, such as land, buildings, machines or intellectual property. An employee’s salary will be recorded as labour income, and their particular wage will be ranked in the labour income distribution. In contrast, any income that is not earned through work but rather through ownership, would be recorded as capital income and computed within the capital share of income.
It is the first time that comprehensive international data on labour income and its distribution has been produced and published. Some existing databases contain data on overall income inequality or consumption inequality, but no data existed concerning the distribution of labour income. We didn’t even know how much labour income was earned globally, much less its detailed distribution for a large set of countries. However, many current debates involving inequality such as automation, the decline of the middle class, or the minimum wage are very plausibly related to factors within the labour market. But, until now we didn’t have a clear picture of the income side of the labour market.
We now have a much clearer picture of the distribution of labour income at the global level, and in particular how low the labour income of the lowest paid workers is. There’s a reason for this: little to no information was available concerning the labour income of the self-employed. Yet, globally they represent almost half of the workforce, or around 1.5 billion workers. This has significant implications for inequality: in the developing world the self-employed tend to face worse working conditions in terms of earnings than employees, so not taking them into account can be highly misleading.
But the issue goes deeper. In low-income countries, employees only represent one-fifth of the workforce, whereas in high-income countries the share is close to 90%. With these huge discrepancies, before this new dataset was published, any extensive international comparison of labour income was not possible. Now we have detailed data at the country level data and can compare them directly.
The key data source for the dataset is the ILO Harmonized Microdata collection, which consists of labour survey microdata. Moreover, in order to produce an estimate of both the labour income share and the labour income distribution, both survey microdata and national accounts data are required. National accounts data in this context refer to having both the gross domestic product and the item compensation of employees in the United Nations Statistical Division (UNSD) repository of national accounts. Requiring worker level observations (microdata) for each country and year is quite stringent, so, naturally, many observations are missing.
Nonetheless, in order to produce consistent time series, as well as global and regional aggregates that are not biased and excessively volatile – due to missing countries – the missing observations have to be estimated. Although there are multiple statistical models and variables used to produce data for missing observations, two main methods are used: model-based extrapolation and imputation. The model-based extrapolation draws from the fact that for a given country if at least one observation in the series has the required data, a statistical extrapolation, based on those observations and additional socioeconomic variables, yields results that are in general with a relatively low margin of error. Imputation, in contrast, relies on statistical modelling on the basis of relevant socioeconomic variables, but no single data point with which to anchor the estimate for a given country is available. Hence, the margin of error of imputed observations is substantially larger than model-based extrapolated observations. Imputed observations are not suitable for country comparisons, yet they are very useful to reduce the bias and uncertainty of the global and regional estimates. This is the reason for their production in the first place.
The analysis is done in terms of the relative distribution, so by definition – since a distribution always has to add up to 100% – if a particular group experiences gains, another has to lose. But the issue is who in the distribution loses and who gains when the top earners experience gains. For instance, consider the following hypothetical. It could be that when the top 5% gains, the lowest earners would also end up increasing their share, with only workers with upper-middle incomes losing. It turns out that empirically, what we observed is that when the top 5% experiences gains, only incomes at the top increase (approximately the top 10%). Whereas the rest of the distribution experiences losses, and particularly in the case of the lowest-earning workers. Therefore, the data points to the following conclusion: relative increases at the top are only very narrowly shared across top earners.
The analysis is done in terms of the relative distribution, so by definition – since a distribution always has to add up to 100% – if a particular group experiences gains, another has to lose. But the issue is who in the distribution loses and who gains when the median earners experience gains. For instance, consider the following hypothetical. It could be that when the median workers gain, the lowest earners would lose, with top earners increasing their share. It turns out that empirically, what we observed is that when the median workers experience gains, only incomes at the top experience decreases (approximately the top 10%). Whereas the rest of the distribution shares the gains, and particularly in the case of the lowest-earning workers. Therefore, the data points to a high complementary in income distribution: relative increases for median workers are widely shared across the distribution, roughly 80% of workers. Only those at the top of the distribution end up experiencing relative losses.
The results find a negative association in relative terms between the top shares and most of the rest, particularly for the lowest earners. Whether the lowest earners can be made better off in level by increasing top incomes, and then having, higher economic growth as a consequence is not ruled out. However, it is made much harder by the distributional effect. In other words, the additional economic growth supposedly induced by increases in top incomes would need to be significantly higher in order for the lowest earners to be made better off than in the absence of the distributional effects.
Economic convergence certainly has a strong potential to reduce global inequality, as shown by the empirical evidence concerning the last 15 years. There are, however, two qualifications worth mentioning. First, for convergence to work it has to happen in a sufficient number of countries (or in large countries). China, having basically reached the world average, will not be able to drive down inequality as it has in the past. India’s convergence still has room to reduce inequality. But, undoubtedly, if high growth would be sustained in the following decade in low-income countries, this would be a key contributor to reducing global inequality. Second, there is also an issue with perspective. Trends at the national level are arguably most relevant for workers. If workers at the lowest end of the distribution have seen their real wages shrink or their share of the national income pie decline, this will be far more relevant to them than changes in global inequality.
Methods
ILO Modelled Estimates (ILOEST database)
Discover the methods behind the ILO’s modelled estimates on labour force statistics (including the working poor), labour productivity, wage growth and labour migration.
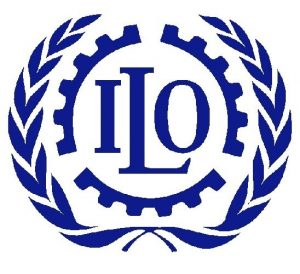
The Global Labour Income Share and Distribution: Methodological Description
The article is organized into four sections. Section II describes in detail the methodology and its relationship to the literature. Section III presents some of the results, including the global labour income share and distribution and their evolution since 2004. Section IV concludes.
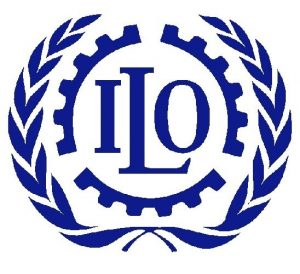
Decent Work Indicators – Guidelines for producers and users of statistical and legal framework indicators
This manual presents guidelines on the decent work statistical indicators and legal framework indicators. It is divided into eleven chapters which correspond to the ten substantive elements of decent work as well as to the economic and social context for decent work.
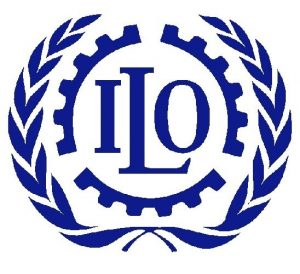
Sources and Methods Volume 6: Household income and expenditure statistics (2003)
This volume presents national methodological descriptions of household income and expenditure statistics derived from various sources, mainly from household surveys. This information containing descriptions for 98 statistical sources was updated in 2003 and is the second edition.
Publications
Note: Many publications are available only in English. If available in other languages, a new page will open displaying these options.

World Employment and Social Outlook: September 2024 Update
This brief focuses on the evolving relationship between labour and capital income, challenges facing youth in the current job market, and progress towards key SDGs as 2030 approaches.

The global labour income share and distribution: Key findings
The global labour income distribution is lopsided: a worker in the top 10% earns US$7,445 (PPP) per month, a worker in the bottom 10% earns just US$22.