The ILO modelled estimates series provides a complete set of internationally comparable labour statistics, including both nationally reported observations and imputed data for countries with missing data. The imputations are produced through a series of econometric models maintained by the ILO. The purpose of estimating labour market indicators for countries with missing data is to obtain a balanced panel data set so that, every year, regional and global aggregates with consistent country coverage can be computed. These allow the ILO to analyse global and regional estimates of key labour market indicators and related trends. Moreover, the resulting country-level data, combining both reported and imputed observations, constitutes a unique, internationally comparable dataset of key labour market indicators.
Estimates for countries with very limited labour market information have a high degree of uncertainty. Hence, estimates for countries with limited nationally reported data should not be considered as “observed” data, and great care needs to be applied when using these data for analysis, especially at the country level.
For more information on the ILO modelled estimates, refer to this:

ILO modelled estimates methodological overview
The ILO modelled estimates series provides a complete set of internationally comparable labour statistics, including both nationally reported observations and imputed data for countries with missing data. The imputations are produced through a series of econometric models maintained by the ILO. This document describes the methodology of the series.
Data collection and evaluation
The ILO modelled estimates are generally derived for 189 countries, disaggregated by sex and age as appropriate. For selected indicators, an additional disaggregation by rural/urban areas is performed. Before running the models to obtain the estimates, labour market information specialists from the ILO Department of Statistics, in cooperation with the Research Department, evaluate existing country‑reported data and select only those observations deemed sufficiently comparable across countries.
The recent efforts by the ILO to produce harmonized indicators from country-reported microdata have greatly increased the comparability of the observations. Nonetheless, it is still necessary to select the data based on the following four criteria: (1) type of data source; (2) geographical coverage; (3) age-group coverage; and (4) presence of methodological breaks or outliers.
Data selection and revision to historical estimates
The ILO maintains a series of econometric models used to produce estimates of labour market indicators for countries and years where country-reported data are unavailable and to produce forecasts (see descriptions below). As in previous years, the ILO modelled estimates have been updated to account for new information. It is important to note that the integration of new information can impact and revise older historical data if newer data are a more trusted type of data source, or it creates methodological breaks. This may lead to the removal of previously included data. Consequently, historical trends reflected in the ILO modelled estimates of November 2024 may be different from those published in November 2023 due to the inclusion of updated data inputs.
An important difference between the ILO modelled estimates of November 2023 and those of November 2024 arises from India’s Periodic Labour Force Survey (PLFS) data. In the November 2023 edition, PLFS data for 2020, 2021, 2022, and the first half of 2023 became available and were included as model inputs, while data from 2018 and 2019 were excluded as they appear to present limited comparability with both the previous NSS results and the newer PLFS results. The PLFS does not strictly follow international labour standards (neither the 13th nor 19th ICLS resolutions), particularly in applying the priority rule, the one-hour employment criterion, and the structured separation of key criteria like job search and availability. Additionally, the reliance on semi-structured interviews and interviewer judgment increases the potential for inconsistencies, reduces transparency and makes the assessment of data quality more difficult. These factors undermine the PLFS’s comparability both with other countries and across different time periods. To ensure that the trends reflected in the estimates are consistent with the broader patterns observed in the NSS and PLFS datasets, the November 2024 edition adopted a revised approach for the labour force participation rates. This approach incorporates NSS and PLFS data points, excluding data from 2018 and 2019, and applies a smoothing function to reduce year-to-year variability and enhance the international comparability of the estimates. Given the country’s size, this has a sizeable impact on the global aggregates.
Country groupings
The UN does not have a standardized set of regional groupings. Groupings in ILOSTAT are based on the regions used for administrative purposes by the ILO, which may differ from those of other organizations. These usually do not change over time.
ILOSTAT also presents income groupings based on the World Bank’s classification. The world’s economies are assigned to one of four income groups: low, lower-middle, upper-middle, and high-income countries. The classifications are updated each year on July 1 and are based on GNI per capita in current USD of the previous year. Aggregates in the ILO modelled estimates from the November edition reflect the World Bank’s income classifications from July that year. Hence, estimates from different editions will not reflect the same income groupings.
Country, territory and area groupings
See the country groupings by ILO region and World Bank income group for data in ILOSTAT. Regional groups vary across UN organizations despite similar names.
F.A.Q.
Conducting labour force surveys is a complicated and costly task which some countries are unable to do on a systematic basis. Consequently, significant data gaps remain in most international labour statistics databases. To be able to produce reliable global and regional estimates of key labour indicators, the ILO has developed statistical models that produce estimates for countries in years for which no data have been reported. These models have been tested for statistical accuracy and allow the ILO to forecast changes in key labour market indicators as well as to produce global and regional aggregates. The end result of these models is a complete set of national labour statistics alongside the global and regional aggregates. In the interest of transparency, the ILO publishes the resulting country-level and global and regional estimates in the ILO modelled estimates series.
Not all countries submit statistically comparable data. Before running the models to obtain the estimates, ILO labour market information specialists evaluate country-reported data and select only those observations deemed sufficiently comparable across countries. The recent efforts by the ILO to produce harmonized indicators from country-reported microdata have greatly increased the comparability of the observations. Nonetheless, it is still necessary to select the data on the basis of the following four criteria: (a) type of data source; (b) geographical coverage; (c) age-group coverage; and (d) presence of methodological breaks or outliers.
Our models also include country-level data on population, economic growth, poverty and other economic indicators from the following sources:
- United Nations World Population Prospects
- IMF/World Bank data on macroeconomic indicators
- World Bank poverty estimates from the PovcalNet database
The estimates are produced using a series of models, which establish statistical relationships between observed labour market indicators and explanatory variables. These relationships are used to impute missing observations and to make projections for the indicators.
There are many potential statistical relationships, also called “model specifications” that could be used to predict labour market indicators. The key to obtaining accurate and unbiased estimates is to select the best model specification in each case. The ILO modelled estimates generally rely on a procedure called cross-validation, which is used to identify those models that minimize the expected error and variance of the estimation. This procedure involves repeatedly computing a number of candidate model specifications using random subsets of the data: the missing observations are predicted and the prediction error is calculated for each iteration. This makes it possible to identify the statistical relationship that provides the best estimate of a given labour market indicator.
The ILO modelled estimates aim is to provide a complete set (without missing observations) of internationally comparable labour statistics. In order to achieve complete and comparable estimates several harmonisation processes are carried out, which can result in estimates differing from nationally reported data. The following procedures are the most common source of differences:
- Benchmarking the working-age population to the estimates of the United Nations World Population Prospects.
- Application of the standards adopted by the International Conference of Labour Statisticians to produce internationally comparable figures.
- Adjustment of classifications for standardization purposes. For instance, adjusting data to account for differences in age coverage.
- Internal consistency procedures. Each edition of the ILO modelled estimates is internally consistent by construction. This entails ensuring that components add up to the total in every observation across all related indicators. Such normalization procedures can produce differences with respect to national sources.
- Mitigation of time series breaks. In order to produce statistics that are comparable over time, in certain instances it is necessary to adjust figures within a time series to account for changes in methodology, coverage or other relevant dimensions.
The ILO modelled estimates cover a wide variety of indicators. Hence, input updates and methodological improvements are implemented in a staggered manner. The time stamp indicates the production date of the estimates, also referred to as the edition. The production date is important because it indicates approximately the cut-off date for inclusion of nationally reported observations as input into the models. Additionally, estimates with the same production date have undergone normalization to ensure that they are internally consistent. For instance, the sum of employment across all economic sectors will equal the sum across all occupations. Nonetheless, for estimates with different production dates, this will not be the case.
We are constantly improving the ILO modelled estimates. Revisions usually happen for one of three reasons:
- Countries make new data available. The ILOSTAT database is constantly updated as new national labour statistics become available. In some cases, this may only happen after a significant delay, requiring the ILO to replace estimates for that year with the reported statistics.
- Revisions are made to other databases used by our statistical model. The ILO’s econometrics models use databases maintained by other international organizations such as the UN’s World Population Prospects and the IMF’s World Economic Outlook. These databases are periodically subject to their own revisions, which can lead to revisions in the ILO modelled estimates.
- Historical data needs to be revised. Periodically, data from prior years needs to be revised as new information emerges.
Please see different options on our dissemination and analysis page.
Labour market indicators
Labour market indicators are estimated using a series of models that establish statistical relationships between observed labour market indicators and explanatory variables. These relationships are used to impute missing observations and to make projections for the indicators.
There are many potential statistical relationships, also called “model specifications”, that could be used to predict labour market indicators. The key to obtaining accurate and unbiased estimates is to select the best model specification in each case. The ILO modelled estimates generally rely on a procedure called “cross-validation”, which is used to identify those models that minimize the expected error and variance of the estimation. This procedure involves repeatedly computing a number of candidate model specifications using random subsets of the data: the missing observations are predicted and the prediction error is calculated for each iteration. Each candidate model is assessed based on the pseudo-out-of-sample root mean square error, although other metrics such as result stability are also assessed depending on the model. This makes it possible to identify the statistical relationship that provides the best estimate of a given labour market indicator. It is worth noting that the most appropriate statistical relationship for this purpose may differ according to country.
The benchmark for the ILO modelled estimates is the 2024 Revision of the United Nations World Population Prospects, which provides estimates and projections of the total population broken down into five-year age groups. The working-age population comprises everyone who is at least 15 years of age. Although the same basic approach is followed in the models used to estimate all the indicators, there are differences between the various models because of specific features of the underlying data. Further details are provided for each model in this methodological description, while an overview is provided below.
Conflict countries
Within the series of econometric models used to produce estimates of labour market indicators in the countries and years for which country-reported data are unavailable and to produce forecasts, the ILO includes an econometric model for countries during years of conflict. The econometric model measures the elasticity of the target variable of interest and employment and GDP per capita (during 2020, a period of severe supply and demand shocks) for all countries with available data. The model then uses these estimated elasticities to reflect changes in the target variable using changes in employment and GDP per capita during conflict years. An example of this methodology applied to Ukraine can be found in the ILO Monitor on the world of work, Tenth edition. Given the exceptional situation, including the scarcity of relevant data, the estimates for countries in years of conflict are subject to exceptionally high uncertainty.
Labour force, employment structure and labour underutilization
To track the participation in the labour market of the working-age population, estimates of the labour force are produced, disaggregated by sex and age. The labour force measures active participation in the labour market: the sum of persons employed and the unemployed. To analyse the employment structure, the distribution of employment as a function of four different breakdowns is estimated: employment status, economic activity (sector), occupation, economic class (working poverty), and informality. To measure labour underutilization, there are numerous series available disaggregated by sex and age: unemployment rate (LU1), labour underutilization rates (LU2 and LU4), the NEET rate (youth not in employment, education or training), time-related underemployment rate, and others. For some of the indicators described a breakdown by rural/urban areas is produced. Projections are only available for selected indicators. Moreover, for the estimates of informality, LU3, and the jobs gap, only regional and global aggregate estimates are available.
Hours worked
Hours worked model
The ILO nowcasting model pertaining to changes in quarterly hours worked during the pandemic has now been replaced with a yearly model of hours worked. This new series of hours related indicators includes total weekly hours worked of employed persons, the ratio of total weekly hours worked to population, mean weekly hours actually worked per employed person, and the number of full-time equivalent jobs.
- Total weekly hours worked by employed persons.
- Mean weekly hours actually worked per employed person.
- Ratio of total weekly hours worked to population aged 15-64.
- Number of full-time equivalent jobs (assuming 40 or 48 workweek hours). This measure is constructed by dividing the total number of weekly hours actually worked by 40 or 48.
Hours worked ILO modelled estimates begin in 2005 and go up to projections for 2025 and 2026.
Labour income
The dataset covers 189 countries as well as global and regional aggregates. The data are based on the ILO Harmonized Microdata collection. To produce consistent time series for all countries, statistical models are used to extrapolate and impute missing data points. The dataset contains two key indicators: the labour income share and the labour income distribution, following the recommendation of the ILO Global Commission on the Future of Work to develop new distributional indicators. Furthermore, the new internationally comparable labour share data will be used to monitor progress towards the United Nations’ Sustainable Development Goals.
Wages
The methodology to estimate global and regional wage trends was developed by the ILO for the previous editions of the Global Wage Report (GWR) in collaboration between technical departments and the Department of Statistics, following four peer reviews conducted by five independent experts. The appendix of the GWR describes the methodology adopted as a result of this process.
Global estimates on wages are not published on ILOSTAT.
Labour migration
The fourth edition of the ILO Global Estimates on International Migrant Workers presents the most recent estimates on the stock of international migrant workers, disaggregated by age, sex, country-income group and region, and the estimation methodology. The reference year is 2022. The report predates the onset of the COVID-19 crisis, which has affected the magnitude and characteristics of international labour migration.
Global estimates on labour migration are not published on ILOSTAT.
Child labour
The current (sixth) edition of the Global Estimates of Child Labour provides updated estimates for 2020 and has been produced for the first time in partnership with UNICEF. The ILO-UNICEF estimates are based on the international standards concerning statistics on child labour, which were adopted by the 20th International Conference of Labour Statisticians (ICLS) in October 2018. These standards outline statistical definitions of child labour and its components, hazardous work by children and the worst forms of child labour other than hazardous work. To gauge trends in child labour and other related indicators at the regional and global levels, a series of econometric models were developed to account for the non-randomness in missing data. These efforts improve the accuracy of the estimates and also ensure replicability of the estimation process, thereby facilitating updates and the development of subsequent global estimates. A report presents the methodological protocols used for the development of the 2020 ILO-UNICEF Global Estimates of Child Labour.
Publications
Note: Many publications are available only in English. If available in other languages, a new page will open displaying these options.

ILO modelled estimates methodological overview
The ILO modelled estimates series provides a complete set of internationally comparable labour statistics, including both nationally reported observations and imputed data for countries with missing data. The imputations are produced through a series of econometric models maintained by the ILO. This document describes the methodology of the series.
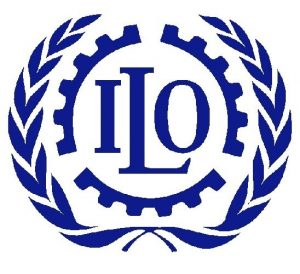
Employment and economic class in the developing world
This paper introduces a model for generating national estimates and projections of the distribution of the employed across five economic classes for 142 developing countries over the period 1991 to 2017. The national estimates are used to produce aggregate estimates for eight developing regions and for the developing world as a whole.
Indicator catalogue
Indicator | Frequency | Database | Subject | Download (with labels) | Download (with codes) | Data explorer |
---|---|---|---|---|---|---|
Population by sex and age -- UN estimates and projections, July 2024 (thousands) | Annual | ILO Modelled Estimates (ILOEST) | Population | .csv .dta | .csv.gz | |
Population by sex, age and rural / urban areas -- UN estimates, July 2024 (thousands) | Annual | ILO Modelled Estimates (ILOEST) | Population | .csv .dta .xlsx | .csv.gz | |
Population by rural / urban areas -- UN estimates and projections, July 2024 (thousands) | Annual | ILO Modelled Estimates (ILOEST) | Population | .csv .dta .xlsx | .csv.gz | |
Labour dependency ratio -- ILO modelled estimates, Nov. 2024 | Annual | ILO Modelled Estimates (ILOEST) | Population | .csv .dta .xlsx | .csv.gz | |
Labour force by sex and age -- ILO modelled estimates, Nov. 2024 (thousands) | Annual | ILO Modelled Estimates (ILOEST) | Labour force | .csv .dta .xlsx | .csv.gz | |
Labour force by sex, age and rural / urban areas -- ILO modelled estimates, Nov. 2021 (thousands) | Annual | ILO Modelled Estimates (ILOEST) | Labour force | .csv .dta .xlsx | .csv.gz | |
Labour force participation rate by sex and age -- ILO modelled estimates, Nov. 2024 (%) | Annual | ILO Modelled Estimates (ILOEST) | Labour force | .csv .dta .xlsx | .csv.gz | |
Labour force participation rate by sex, age and rural / urban areas -- ILO modelled estimates, Nov. 2021 (%) | Annual | ILO Modelled Estimates (ILOEST) | Labour force | .csv .dta .xlsx | .csv.gz | |
Employment by sex and age -- ILO modelled estimates, Nov. 2024 (thousands) | Annual | ILO Modelled Estimates (ILOEST) | Employment | .csv .dta .xlsx | .csv.gz | |
Employment by sex, age and rural / urban areas -- ILO modelled estimates, Nov. 2021 (thousands) | Annual | ILO Modelled Estimates (ILOEST) | Employment | .csv .dta .xlsx | .csv.gz | |
Employment by sex and status in employment -- ILO modelled estimates, Nov. 2024 (thousands) | Annual | ILO Modelled Estimates (ILOEST) | Employment | .csv .dta .xlsx | .csv.gz | |
Employment by age and status in employment -- ILO modelled estimates, Nov. 2024 (thousands) | Annual | ILO Modelled Estimates (ILOEST) | Employment | .csv .dta .xlsx | .csv.gz | |
Employment by sex, rural / urban areas and status in employment -- ILO modelled estimates, Nov. 2021 (thousands) | Annual | ILO Modelled Estimates (ILOEST) | Employment | .csv .dta .xlsx | .csv.gz | |
Employment by sex and economic activity -- ILO modelled estimates, Nov. 2024 (thousands) | Annual | ILO Modelled Estimates (ILOEST) | Employment | .csv .dta .xlsx | .csv.gz | |
Employment by sex, rural / urban areas and economic activity -- ILO modelled estimates, Nov. 2020 (thousands) | Annual | ILO Modelled Estimates (ILOEST) | Employment | .csv .dta .xlsx | .csv.gz | |
Employment by sex and occupation -- ILO modelled estimates, Nov. 2024 (thousands) | Annual | ILO Modelled Estimates (ILOEST) | Employment | .csv .dta .xlsx | .csv.gz | |
Employment by sex, rural / urban areas and occupation -- ILO modelled estimates, Nov. 2020 (thousands) | Annual | ILO Modelled Estimates (ILOEST) | Employment | .csv .dta .xlsx | .csv.gz | |
Employment by sex, age and economic class -- ILO modelled estimates, Nov. 2024 (thousands) | Annual | ILO Modelled Estimates (ILOEST) | Working poverty | .csv .dta .xlsx | .csv.gz | |
Informal employment by sex -- ILO modelled estimates, Nov. 2024 (thousands) | Annual | ILO Modelled Estimates (ILOEST) | Informal economy | .csv .dta .xlsx | .csv.gz | |
Informal employment rate by sex -- ILO modelled estimates, Nov. 2024 (%) | Annual | ILO Modelled Estimates (ILOEST) | Informal economy | .csv .dta .xlsx | .csv.gz | |
Employment-to-population ratio by sex and age -- ILO modelled estimates, Nov. 2024 (%) | Annual | ILO Modelled Estimates (ILOEST) | Employment | .csv .dta .xlsx | .csv.gz | |
Employment-to-population ratio by sex, age and rural / urban areas -- ILO modelled estimates, Nov. 2021 (%) | Annual | ILO Modelled Estimates (ILOEST) | Employment | .csv .dta .xlsx | .csv.gz | |
Time-related underemployment by sex and age -- ILO modelled estimates, Nov. 2022 (thousands) | Annual | ILO Modelled Estimates (ILOEST) | Time-related underemployment | .csv .dta .xlsx | .csv.gz | |
Time-related underemployment by sex, age and rural / urban areas -- ILO modelled estimates, Nov. 2020 (thousands) | Annual | ILO Modelled Estimates (ILOEST) | Time-related underemployment | .csv .dta .xlsx | .csv.gz | |
Time-related underemployment rate by sex and age -- ILO modelled estimates, Nov. 2022 (%) | Annual | ILO Modelled Estimates (ILOEST) | Time-related underemployment | .csv .dta .xlsx | .csv.gz | |
Unemployment by sex and age -- ILO modelled estimates, Nov. 2024 (thousands) | Annual | ILO Modelled Estimates (ILOEST) | Unemployment | .csv .dta .xlsx | .csv.gz | |
Unemployment by sex, age and rural / urban areas -- ILO modelled estimates, Nov. 2021 (thousands) | Annual | ILO Modelled Estimates (ILOEST) | Unemployment | .csv .dta .xlsx | .csv.gz | |
Unemployment rate by sex and age -- ILO modelled estimates, Nov. 2024 (%) | Annual | ILO Modelled Estimates (ILOEST) | Unemployment | .csv .dta .xlsx | .csv.gz | |
Unemployment rate by sex, age and rural / urban areas -- ILO modelled estimates, Nov. 2021 (%) | Annual | ILO Modelled Estimates (ILOEST) | Unemployment | .csv .dta .xlsx | .csv.gz | |
Combined rate of time-related underemployment and unemployment (LU2) by sex and age -- ILO modelled estimates, Nov. 2022 (%) | Annual | ILO Modelled Estimates (ILOEST) | Other measures of labour underutilization | .csv .dta .xlsx | .csv.gz | |
Combined rate of time-related underemployment and unemployment (LU2) by sex, age and rural / urban areas -- ILO modelled estimates, Nov. 2020 (%) | Annual | ILO Modelled Estimates (ILOEST) | Other measures of labour underutilization | .csv .dta .xlsx | .csv.gz | |
Combined rate of unemployment and potential labour force (LU3) by sex -- ILO modelled estimates, Nov. 2024 (%) | Annual | ILO Modelled Estimates (ILOEST) | Other measures of labour underutilization | .csv .dta .xlsx | .csv.gz | |
Combined rate of unemployment and potential labour force (LU3) by sex, age and rural / urban areas -- ILO modelled estimates, Nov. 2020 (%) | Annual | ILO Modelled Estimates (ILOEST) | Other measures of labour underutilization | .csv .dta .xlsx | .csv.gz | |
Composite measure of labour underutilization (LU4) by sex and age -- ILO modelled estimates, Nov. 2022 (thousands) | Annual | ILO Modelled Estimates (ILOEST) | Other measures of labour underutilization | .csv .dta .xlsx | .csv.gz | |
Composite rate of labour underutilization (LU4) by sex and age -- ILO modelled estimates, Nov. 2022 (%) | Annual | ILO Modelled Estimates (ILOEST) | Other measures of labour underutilization | .csv .dta .xlsx | .csv.gz | |
Composite rate of labour underutilization (LU4) by sex, age and rural / urban areas -- ILO modelled estimates, Nov. 2020 (%) | Annual | ILO Modelled Estimates (ILOEST) | Other measures of labour underutilization | .csv .dta .xlsx | .csv.gz | |
Jobs gap by sex -- ILO modelled estimates, Nov. 2024 (thousands) | Annual | ILO Modelled Estimates (ILOEST) | Other measures of labour underutilization | .csv .dta .xlsx | .csv.gz | |
Jobs gap rate by sex -- ILO modelled estimates, Nov. 2024 (%) | Annual | ILO Modelled Estimates (ILOEST) | Other measures of labour underutilization | .csv .dta .xlsx | .csv.gz | |
Potential labour force by sex -- ILO modelled estimates, Nov. 2024 (thousands) | Annual | ILO Modelled Estimates (ILOEST) | Other measures of labour underutilization | .csv .dta .xlsx | .csv.gz | |
Potential labour force by sex, age and rural / urban areas -- ILO modelled estimates, Nov. 2020 (thousands) | Annual | ILO Modelled Estimates (ILOEST) | Other measures of labour underutilization | .csv .dta .xlsx | .csv.gz | |
Potential labour force rate by sex -- ILO modelled estimates, Nov. 2024 (%) | Annual | ILO Modelled Estimates (ILOEST) | Other measures of labour underutilization | .csv .dta .xlsx | .csv.gz | |
Potential labour force and willing non-jobseekers by sex -- ILO modelled estimates, Nov. 2024 (thousands) | Annual | ILO Modelled Estimates (ILOEST) | Other measures of labour underutilization | .csv .dta .xlsx | .csv.gz | |
Potential labour force and willing non-jobseekers rate -- ILO modelled estimates, Nov. 2024 (%) | Annual | ILO Modelled Estimates (ILOEST) | Other measures of labour underutilization | .csv .dta .xlsx | .csv.gz | |
Persons outside the labour force by sex and age -- ILO modelled estimates, Nov. 2024 (thousands) | Annual | ILO Modelled Estimates (ILOEST) | Other measures of labour underutilization | .csv .dta .xlsx | .csv.gz | |
Youth not in employment, education or training (NEET) by sex -- ILO modelled estimates, Aug. 2024 (thousands) | Annual | ILO Modelled Estimates (ILOEST) | Other measures of labour underutilization | .csv .dta .xlsx | .csv.gz | |
Output per hour worked (GDP constant 2021 international $ at PPP) -- ILO modelled estimates, Nov. 2024 | Annual | ILO Modelled Estimates (ILOEST) | Labour productivity | .csv .dta .xlsx | .csv.gz | |
Youth not in employment, education or training (NEET) by sex and rural / urban areas -- ILO modelled estimates, Nov. 2020 (thousands) | Annual | ILO Modelled Estimates (ILOEST) | Other measures of labour underutilization | .csv .dta .xlsx | .csv.gz | |
Inactivity rate by sex and age -- ILO modelled estimates, Nov. 2024 (%) | Annual | ILO Modelled Estimates (ILOEST) | Other measures of labour underutilization | .csv .dta .xlsx | .csv.gz | |
Inactivity rate by sex, age and rural / urban areas -- ILO modelled estimates, Nov. 2021 (%) | Annual | ILO Modelled Estimates (ILOEST) | Other measures of labour underutilization | .csv .dta .xlsx | .csv.gz | |
Share of youth not in employment, education or training (NEET) by sex -- ILO modelled estimates, Aug. 2024 (%) | Annual | ILO Modelled Estimates (ILOEST) | Other measures of labour underutilization | .csv .dta .xlsx | .csv.gz | |
Share of youth not in employment, education or training (NEET) by sex and rural / urban areas-- ILO modelled estimates, Nov. 2020 (%) | Annual | ILO Modelled Estimates (ILOEST) | Other measures of labour underutilization | .csv .dta .xlsx | .csv.gz | |
Labour income share as a percent of GDP -- ILO modelled estimates, May 2025 (%) | Annual | ILO Modelled Estimates (ILOEST) | Labour income | .csv .dta .xlsx | .csv.gz | |
Labour income distribution -- ILO modelled estimates, Nov. 2022 (%) | Annual | ILO Modelled Estimates (ILOEST) | Labour income | .csv .dta .xlsx | .csv.gz | |
Output per worker (GDP constant 2021 international $ at PPP) -- ILO modelled estimates, Nov. 2024 | Annual | ILO Modelled Estimates (ILOEST) | Labour productivity | .csv .dta .xlsx | .csv.gz | |
Gross Domestic Product (GDP constant 2021 international $ at PPP) -- ILO modelled estimates, Nov. 2024 (millions of 2021 PPP dollars) | Annual | ILO Modelled Estimates (ILOEST) | Labour productivity | .csv .dta .xlsx | .csv.gz | |
Gender income gap, ratio of women's to men's labour income -- ILO modelled estimates, May 2025 (%) | Annual | ILO Modelled Estimates (ILOEST) | Labour income | .csv .dta .xlsx | .csv.gz | |
Output per worker (GDP constant 2015 US $) -- ILO modelled estimates, Nov. 2024 | Annual | ILO Modelled Estimates (ILOEST) | Labour productivity | .csv .dta .xlsx | .csv.gz | |
Prime-age labour force participation rate by sex and household type -- ILO modelled estimates, Nov. 2023 (%) | Annual | ILO Modelled Estimates (ILOEST) | Labour force | .csv .dta .xlsx | .csv.gz | |
Prime-age labour force by sex and household type -- ILO modelled estimates, Nov. 2023 (thousands) | Annual | ILO Modelled Estimates (ILOEST) | Labour force | .csv .dta .xlsx | .csv.gz | |
Full-time equivalent employment by sex -- ILO modelled estimates, Nov. 2024 (thousands) | Annual | ILO Modelled Estimates (ILOEST) | Employment | .csv .dta .xlsx | .csv.gz | |
Ratio of total weekly hours worked to population aged 15-64, by sex -- ILO modelled estimates, Nov. 2024 | Annual | ILO Modelled Estimates (ILOEST) | Hours of work | .csv .dta .xlsx | .csv.gz | |
Total weekly hours worked of employed persons, by sex -- ILO modelled estimates, Nov. 2024 (thousands) | Annual | ILO Modelled Estimates (ILOEST) | Hours of work | .csv .dta .xlsx | .csv.gz | |
Mean weekly hours actually worked per employed person by sex -- ILO modelled estimates, Nov. 2024 | Annual | ILO Modelled Estimates (ILOEST) | Hours of work | .csv .dta .xlsx | .csv.gz |