“Break the bias” was the theme of International Women’s Day this year, which focused attention on the persistent biases, stereotypes and discrimination that hold society back from being inclusive and gender equal. When it comes to statistics on the world of work, household surveys remain the best way to obtain unbiased information from a random sample of the population. As such, survey data, the methods used for its collection and the statistics ultimately produced, have a central role to play in breaking gender bias.
There are two pathways through which bias can undermine the utility of survey data for gender-based analysis. First, bias in terms of statistical error relating to how adequately a survey sample corresponds to the population of interest, and how adequately statistical concepts correspond to the subjects, objects, and phenomena they represent. Second, bias in terms of conceptual blind spots and omissions, which relates to who and what is (and is not) designated as eligible for representation and measurement in official statistics.
Gender bias imported through statistical error
Sample surveys aim to generate statistics for a population based on values obtained for a subset of sampled individuals (or other sampled units). Statistical error introduced at one or more stages of a survey’s design, administration or processing can threaten the accuracy of the resulting data.1Statistical bias refers to sources of statistical error that are systematic as opposed to random. It can be formally defined as: “an effect which deprives a statistical result of representativeness by systematically distorting it” This definition, adopted by the United Nations Statistics Division in its 2012 glossary, is based on Yadolah Dodge, ed., The Oxford Dictionary of Statistical Terms (Oxford: Oxford University Press, 2003). The elimination of statistical bias (systematic error) – and the measurement and control of variance (random error) is an essential feature of sample survey statistics.
Some level of random sampling error occurs whenever a sample of the population, rather than the entire population of interest, is surveyed. Representative sample survey procedures are designed to minimise and control for this.
Non-sampling error – which may be imported at any, or all, stages of the survey process through coverage error, non-response error and measurement error – is less straightforward to address, as it takes several forms:2Additionally, non-sampling error may originate in processing error and model assumption error, (potentially) arising during data entry, data editing, post-coding, imputation and weighting, and data analysis.
Unit non-response error occurs when it is difficult to access the sampled respondents or respondents are unwilling to participate in a survey. Unit non-response tends to be a more pressing concern in high-income countries, where survey response rates have been in decline for several decades.
Measurement error occurs when the response provided or recorded diverges from the “true” value. Such error can be introduced via the questionnaire, the respondent, the interviewer, the interview context or the survey mode.
Gender bias can be found in each of these error components, though it is particularly relevant to measurement error. Gender bias can be imported through measurement error originating in instrument design (through problems with construct validity, or the wording or sequencing of questions and response codes), survey mode effects, interviewers’ manner (or demographic characteristics), the interview context (for example, respondent self-censorship in the presence of other household members), or insufficient / absent restrictions on proxy-reporting for relevant topics.4Proxy-reporting refers the practice of completing individual-level questionnaire items for eligible household members who are unavailable for interview by requesting that the head of household or another knowledgeable household member provide the information. The permissibility of proxy-reporting depends on the extent to which imperfect information sharing among household members and/or response bias are known or deemed likely to distort reporting. See, for instance: United Nations, Guidelines for Producing Statistics on Asset Ownership from a Gender Perspective, 2019.
Efforts to minimize gender bias in these different sources of statistical error have led to a number of key insights. Methods have evolved to identify, quantify, and classify measurement error at the survey design stage. These include qualitative or “formative” studies, cognitive interviewing, randomized experiments, repeated measurement studies and record-check studies. In some contexts, a move to conversational interviewing has been shown to reduce biases originating in misalignment between respondent comprehension and question intent.
Bias due to blind spots or omissions
As recent works such as Data Feminism and Invisible Women explore, stereotypes depicting distinct social roles, and related spheres of activity, for men and women continue to bias what gets measured, counted, and made visible in statistics.
The effects of such bias have been long noted in the field of labour statistics and have been the subject of extensive corrective efforts. Examples include the undercounting of women engaged in work historically coded as “men’s work” (for instance, labour migration5See UNESCO Office in New Delhi, “Internal Migration in India Initiative”, 2013; ILO, ILO Global Estimates on Migrant Workers: Results and Methodology, 2015. ), and the omission of productive activities historically coded as “women’s work”, such as home-based employment6Termed “home work”, See for instance, International Labour Organisation, Working from home: From invisibility to decent work, 2021. and unpaid care and domestic work. Although vital and valuable (consider the costs to outsource it), most unpaid care and domestic work continues to be excluded from standard measures of economic production, such as gross domestic product.7Efforts to measure the economic value of unpaid household service work through the construction of “extended accounts” (formerly “satellite accounts”) have gained traction in recent years but remain limited in scope and uptake. See United Nations, Guide on Valuing Unpaid Household Service Work, 2018.
These measurement issues and others – together with the scarcity of sex-disaggregated and gender‑relevant data – prompted the establishment of the field of gender statistics in the mid-1980s. Since then, there have been growing efforts to identify and address sources of gender bias in survey statistics. However, as noted by the Commission on the Status of Women at its most recent session earlier this year, work remains to be done in traditional areas of statistics as well as in emerging topics, such as gender data relevant to addressing climate change.8United Nations Economic and Social Council, Achieving gender equality and the empowerment of all women and girls in the context of climate change, environmental and disaster risk reduction policies and programmes, E/CN.6/2022/3, report considered by the Commission on the Status of Women at its 66th session, 14–25 March 2022. 9The ILO has recently highlighted the essential role played by gender statistics in ensuring that climate action provides opportunities for both women and men. See ILO, “Just Transition: An Essential Pathway to Achieving Gender Equality and Social Justice”, 2022.
The ILO’s contribution to breaking the gender bias in statistics
A major advance in overcoming the gender bias in work, employment and labour statistics occurred in 2013, when the highest decision-making body on international labour statistics – the International Conference of Labour Statisticians – adopted a new and expansive reference concept of “work”. This marked an important ‘first,’ and it signified a major step change in the measurement of unpaid productive activities. One important outcome has been the potential for greatly improved analysis of gender-based inequalities in labour force participation, employment characteristics, the division of paid and unpaid labour, and total working time. The ILO is supporting improvements in traditional methods for measuring such gender-related concerns. This includes piloting new light time-use modules for attachment to labour force surveys in low- and middle-income countries, to generate statistics on unpaid care and domestic work when a dedicated time-use survey is not feasible. The ILO is also piloting new labour force survey questions to produce more and better data and statistics on women and men undertaking informal work, the source of paid work for more than 60 per cent of employed people worldwide.
These two pilot projects are linked by their shared goal to reduce gender biases in measures of work. Disproportionate responsibility for the provision of unpaid care and domestic work directly impacts women’s capacity and opportunity to engage in paid work – whether formal or informal.
Learn more
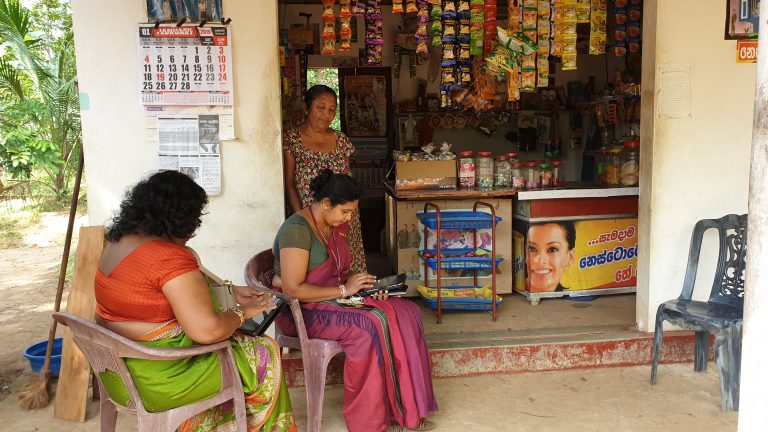
LFS research and development
Learn more about the ILO’s programme of methodological research to identify and promote good practices in the collection and reporting of labour statistics.
The demand for data on unpaid care and domestic work as well as on informal work is growing. There is increasing recognition that such data are necessary to highlight opportunities for transitions to less vulnerable forms of work, and to enable gender-based inequalities to be recognized, reduced, and redistributed.
The methodological research and development work currently being undertaken by the ILO and its partners is essential in supporting countries to produce unbiased and gender-sensitive statistics on the issues that matter most. Survey methods need to be tailored to the measurement challenges faced by developing and emerging economies. Collecting information on time-use in societies where life is less anchored to the 24-hour clock is a case in point. Similarly, challenges exist when asking about unpaid and/or informal work in settings where such activities are not perceived as “work” or there is reluctance to report it.
In his statement to mark International Women’s Day in 2022, the Secretary-General of the United Nations, António Guterres, called for a reversal of power relations and massive investments in training and decent work for women. In the same week, the ILO released its Care at Work report, which finds that up to 300 million new jobs could be created by investing in universal, publicly funded childcare and long-term care services, of which 84 per cent might be formal. High‑quality and unbiased data underpin the transformative work needed to achieve these ambitious objectives. The data are in turn underpinned by robust statistical methodologies. The ILO continues to work closely with countries and other partners to define and operationalize robust statistical standards and support national statistical offices in their application.
Authors
-
Samantha Watson
Samantha is a senior statistician in the ILO Department of Statistics’ Statistical Standards and Methods Unit.
View all posts -
Jessica Gardner
Jessica has worked on improving gender statistics in Asia and the Pacific, Europe, and Central Asia since 2005. She joined the ILO in early 2021 to manage the Engendering Informality Statistics Project, which is being led by the ILO in partnership with the Bill and Melinda Gates Foundation.
View all posts